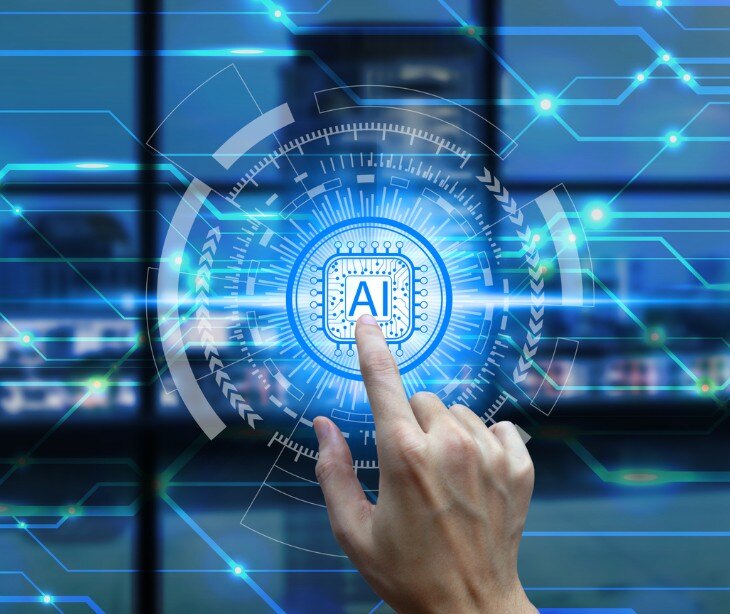
AI hallucinations occur when an artificial intelligence model generates incorrect, misleading, or nonsensical outputs that are not grounded in reality or the input provided.
How do AI hallucinations occur?
These hallucinations can arise in various contexts, such as:
Text generation
- Definition: The AI provides confidently incorrect or fabricated information when asked a question or tasked with generating content.
- Example: The AI invents a fake reference, like a study or article that doesn't exist, or makes up details about a real-world topic.
Image generation
- Definition: The AI creates visual outputs that are distorted, inconsistent, or unrelated to the original prompt.
- Example: Generating an image of a dog with extra legs or merging unrelated objects in a way that doesn't make sense.
Speech and audio
- Definition: AI models produce incoherent or meaningless speech when tasked with audio synthesis or transcription.
- Example: A speech-to-text AI inaccurately transcribes audio in ways that deviate significantly from what was said.
Read also: What is natural language processing?
Why do hallucinations happen?
- Training data limitations: AI models are only as good as the data they are trained on, and gaps or biases in this data can lead to errors.
- Overgeneralization: The model tries to predict or generate something beyond its training or reasoning capabilities.
- Ambiguity in input: Vague, incomplete, or ambiguous prompts can confuse the AI, leading to flawed outputs.
- Optimization trade-offs: AI is often designed to prioritize fluency or coherence over factual accuracy, especially in generative models.
Implications of AI Hallucinations
The consequences of AI hallucinations include:
- Spread of misinformation: If users rely on AI-generated outputs without verification, inaccuracies can propagate widely.
- Loss of trust: Repeated errors undermine user confidence in AI tools, especially in critical domains like healthcare, law, or education.
- Ethical risks: Misleading outputs in sensitive areas, such as medical advice or legal analysis, can have serious consequences.
Addressing AI hallucinations
To address these hallucinations, here are a few things to consider:
Enhancing training data
- Including diverse, high-quality, and updated datasets during training.
Improving model design
- Developing systems that prioritize factual accuracy over fluency when required.
- Incorporating mechanisms to flag uncertainty or low-confidence outputs.
User awareness and verification
- Educating users to critically evaluate AI-generated outputs.
- Encouraging users to cross-check information, especially for high-stakes decisions.
Real-time feedback
- Allowing users to provide feedback to correct hallucinations, helping to refine future responses.
See also: HIPAA Compliant Email: The Definitive Guide
FAQs
Are all AI systems prone to hallucinations?
Yes, all AI systems, especially generative models, are susceptible to hallucinations. However, the likelihood and severity depend on:
- The model’s design and training.
- The complexity of the task or query.
- The domain of application (e.g., scientific writing vs. casual conversation).
Will future AI systems eliminate hallucinations entirely?
It’s unlikely that hallucinations will be entirely eliminated, but ongoing advancements aim to significantly reduce their frequency and impact. Users should continue to verify outputs and use AI responsibly, even as systems improve