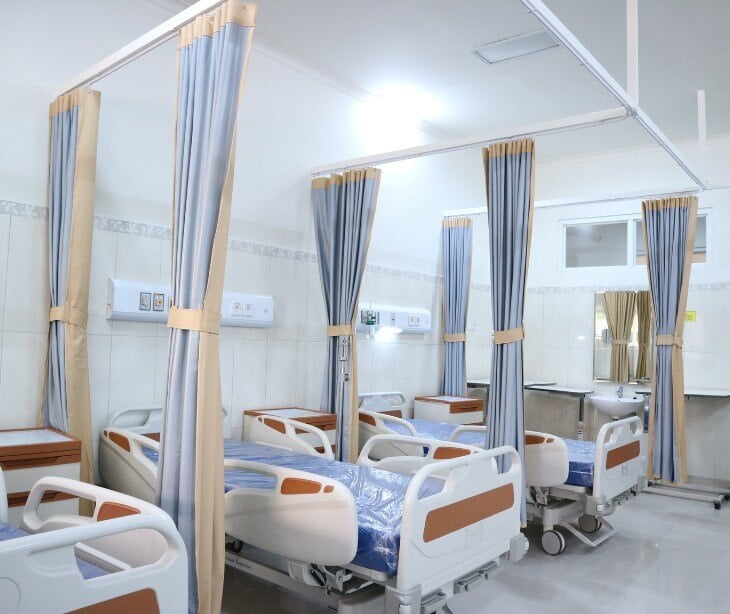
Predictive analytics is an analytical process that leverages historical data to forecast future events, especially those in the healthcare sector. The use of techniques like data mining, machine learning, and statistical modeling can improve patient flow management. Through the understanding of patterns, healthcare facilities can proactively adjust staffing levels, and optimize bed assignments and accommodations.
The data sources used for predictive analytics
- Electronic health records (EHR): These contain comprehensive patient medical histories, including diagnoses, treatments, and medications.
- Insurance claims data: This information provides insights into patient demographics, treatment patterns, and costs associated with healthcare services.
- Laboratory results: Data from lab tests helps in assessing patient health and predicting potential health risks.
- Radiology images: Imaging data such as X-rays and MRIs can be analyzed for diagnostic purposes and to identify trends in patient conditions.
- Wearable devices: Data from fitness trackers and smartwatches contribute real-time health metrics like heart rate and activity levels.
- Patient surveys and feedback: Information gathered from patient experiences can help identify areas for improvement in care delivery.
- Social determinants of health: Data on factors like socioeconomic status, education, and environment can provide context for patient health outcomes.
- Clinical trials data: Results from clinical studies contribute to understanding treatment efficacy and patient responses.
How machine learning techniques are used
Machine learning involves the development of algorithms that can learn from and make predictions based on data. Machines are able to analyze vast amounts of medical data, from EHRs to imaging, to identify patterns that may not be immediately apparent to human practitioners.
According to a study published in Current Genomics, “Current machine learning advancements in healthcare have primarily served as a supportive role in a physician or analyst's ability to fulfill their roles, identify healthcare trends, and develop disease prediction models.”
Predictive analysis powered by machine learning can forecast patient flow and health risks which allows providers to manage resources and interventions proactively. Algorithms can analyze historical patient data to predict which patients are at risk of readmission or complications. It serves as a preemptive measure to improve patient care.
The uses of predictive analytics in patient flow management
- Early detection of disease outbreaks: Predictive analytics can analyze historical health data and social determinants to forecast potential outbreaks of infectious diseases. Identifying patterns in patient visits and lab results helps implement preventive measures and allocate resources effectively before an outbreak escalates.
- Personalized treatment plans: Using genetic, environmental, and lifestyle data, predictive analytics can help create treatment plans for individual patients. The approach optimizes medication effectiveness and minimizes adverse reactions by predicting how different patients will respond to specific treatments.
- Predicting patient readmissions: Healthcare facilities can use predictive models to identify patients at high risk of readmission based on their medical history, demographics, and social factors. Providers can reduce readmission rates and improve patient outcomes by targeting these individuals with follow-up care and interventions.
- Optimizing surgical scheduling: Predictive analytics can enhance surgical scheduling by analyzing historical data on surgery durations, recovery times, and patient flow. Optimization ensures that operating rooms are used efficiently.
- Resource allocation in emergency departments: In emergency departments, predictive analytics can forecast patient volumes based on time of day, day of the week, and seasonal trends. Forecasting allows for better staffing decisions and resource allocation during peak times.
- Identifying high-risk patients for chronic disease management: Healthcare providers can use predictive analytics to identify patients at risk for chronic diseases such as diabetes or heart disease. Analyzing factors like lifestyle choices and family history, interventions can be implemented early to prevent disease progression.
Related: HIPAA Compliant Email: The Definitive Guide
FAQs
What is care coordination?
Care coordination involves organizing patient care activities and sharing information among all participants concerned with a patient's care to facilitate appropriate delivery of services.
How is patient data managed in healthcare?
Patient data is collected, stored, and analyzed using electronic health records (EHRs) and patient management software to ensure accuracy and accessibility for informed decision-making.
What is patient management?
Patient management refers to the coordinated approach of overseeing and delivering healthcare services to patients, ensuring they receive appropriate and timely care.