4 min read
De-identification techniques impact for clinical care and research
Kirsten Peremore
Mar 30, 2025 7:16:04 AM
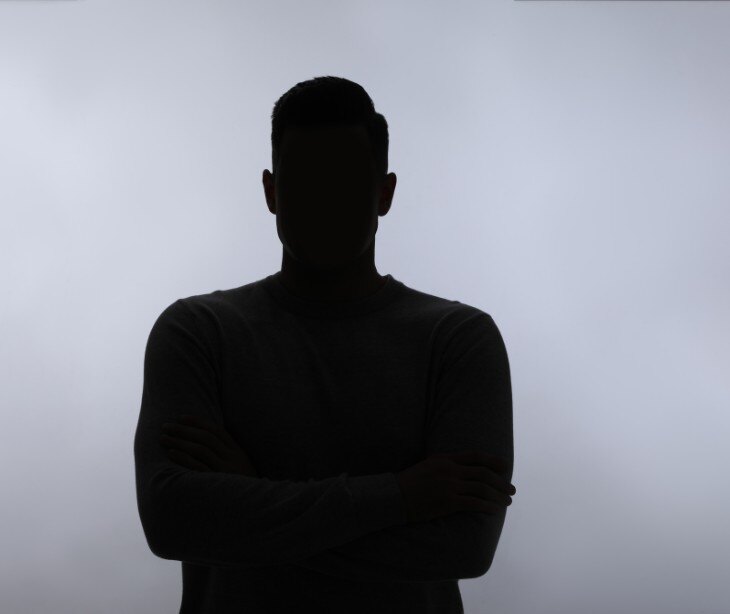
Ultimately, de-identification remains a double-edged sword as it is necessary for privacy compliance, but is not a standalone solution. In clinical care, the Safe Harbor method is one often used to strip datasets of granular details needed for treatment coordination. An AMIA study titled ‘Modes of De-identification’ notes, “If dates, ages over 89 years, and/or detailed geographic information (five-digit ZIP code or at the town level of details) are required in the study, Safe Harbor method can be used along with another HIPAA Privacy Rule provision called Limited Data Set.” While it protects against breaches, it introduces operational bottlenecks when re-identification is necessary for continuity of care, as seen in cases where providers must temporarily revert pseudonymized records to access full patient histories.
For research, deidentification enables large-scale data sharing across institutions, fostering advancements in public health and drug development. However, over-generalization (e.g., broad age ranges) or synthetic data generation may dilute dataset accuracy. Reidentification risks persist through "quasi-identifiers", combinations of seemingly harmless data points (e.g., ZIP code + rare diagnosis) that can unmask individuals when cross-referenced with external datasets.
HIPAA’s lack of a quantified "very small" re-identification risk threshold under Expert Determination introduces subjectivity, leaving gaps for exploitation via AI-driven linkage attacks.
The methods of deidentification regulated by HIPAA
Deidentification is the removal or alteration of protected health information (PHI) to ensure it cannot reasonably identify an individual. The process makes the data exempt from the Privacy Rule restrictions attached to PHI. Defined in 45 CFR §164.514, it also aims to balance privacy preservation with data utility for research and public health. There are two main methods under HIPAA: the Safe Harbor and Expert Determination Methods.
Safe Harbor Method
The Safe Harbor approach requires the removal of 18 specific identifiers from PHI, including names, geographic subdivisions smaller than a state, exact dates (except year), contact information, biometric data, and unique identifiers like Social Security or medical record numbers. According to a collaborative study titled ‘Re-identification Risks in HIPAA Safe Harbor Data: A study of data from one environmental health study’, “The HIPAA Safe Harbor standard uses a traditional pillar of data privacy known as deidentification – the removal of explicit identifiers from data to make the result sufficiently anonymous. The rationale behind de-identification is simple. If an individual cannot be distinctly identified in data, then no individual’s privacy interests are affected, so the data can be shared widely for many worthy purposes.”
Expert Determination Method
This method allows retention of some identifiers if a qualified expert statistically validates that re-identification risk is “very small” when used alone or combined with “reasonably available” external data. Experts apply techniques like generalization, perturbation, or k-anonymity, tailoring de-identification to the dataset’s context. According to ‘Guidance Regarding Methods for De-identification of Protected Health Information in Accordance with the Health Insurance Portability and Accountability Act (HIPAA) Privacy Rule’ the central challenge with this method is that “There is no specific professional degree or certification program for designating who is an expert at rendering health information de-identified.” HIPAA’s vague “very small” risk threshold introduces subjectivity. The rule does not define measurable benchmarks (e.g., probability limits), leaving experts to interpret risk based on evolving re-identification tactics.
Understanding patient identification
Accurate patient identification is a way to ensure that care is delivered to the correct individual. The Joint Commission emphasizes the use of two patient identifiers before providing care.
The Joint Commission states that the glossary of the accreditation manual notes, “Information directly associated with an individual that reliably identifies the individual as the person for whom the service or treatment is intended. Acceptable identifiers may be the individual's name, an assigned identification number, telephone number, date of birth or other person-specific identifier.”
This dual-identifier approach helps prevent mix-ups and ensures that treatments are matched to the intended patient. However, when data is deidentified for research or other purposes, these identifiers must be removed or modified to prevent reidentification, as per HIPAA's Safe Harbor or Expert Determination methods.
The risks presented by deidentification
Despite deidentification efforts, studies have shown that patient data remains vulnerable to reidentification when quasi-identifiers, such as ZIP codes or rare medical conditions, are cross-referenced with external datasets. A study about re-identification of patients revealed that 28.3% of individuals in Maine and 34% in Vermont were successfully re-identified from hospital data that adhered to HIPAA's Safe Harbor guidelines. Similarly, research from The Canadian Journal of Hospital Pharmacy on prescription records found that without deidentification algorithms, the probability of reidentification was high due to the uniqueness of certain data points like admission dates and laboratory results.
These risks are exacerbated by advancements in artificial intelligence and data analytics, which can exploit patterns in ostensibly anonymized datasets to uncover individual identities. An attacker using just 5–7 laboratory results from a patient could identify their corresponding record in a de-identified biomedical database. These breaches compromise patient confidentiality and expose sensitive health information that could be misused for discrimination. While some studies argue that the risk of re-identification from publicly available health data is low compared to large-scale data breaches, the lack of universal thresholds for acceptable risk levels leaves healthcare organizations vulnerable to privacy violations.
How deidentification impacts patient identification procedures
The reasonable impact of deidentification on patient identification processes lies in its ability to create operational inefficiencies and data fragmentation. Patient identification relies on precise information like medical record numbers or demographic details to ensure that treatments are matched to the correct individual. According to a Learning Health Systems policy, “When prospective informed consent is not a viable or desirable option, the return of results to patients could support transparency regarding secondary data use. However, deidentification renders the return of results difficult or impossible.”
When these identifiers are removed or generalized through de-identification techniques, healthcare providers may face delays in accessing complete patient histories or reconciling records across systems. It is particularly problematic in cases where re-identification is necessary, for instance, when linking de-identified research data back to a specific patient for follow-up care. These procedures require additional administrative steps and access controls, which can strain resources and workflows within healthcare organizations.
FAQs
What are the common methods of deidentification?
- Suppression: Removing identifiers entirely (e.g., names, SSNs).
- Generalization: Replacing specifics with broader categories (e.g., age ranges).
- Perturbation: Adding noise to disrupt individual data points.
- Tokenization/pseudonymization: Replacing identifiers with reversible codes.
- Synthetic data generation: Creating artificial datasets mimicking real trends.
When is deidentification necessary in clinical settings?
Deidentification is necessary in clinical settings when data needs to be shared with external parties, such as researchers or third-party vendors. It is also required when data is used for secondary purposes, such as quality improvement initiatives or public health studies.
What triggers reidentification?
Reidentification can be triggered by the combination of quasi-identifiers (e.g., age, location, rare diagnoses) with external datasets, allowing individuals to be identified despite initial deidentification efforts. Advanced computational methods, including AI-driven linkage attacks, can also facilitate re-identification by analyzing patterns across multiple datasets.
Can deidentified data be shared without consent?
Generally, deidentified data can be shared without consent, but it must meet specific standards.
What triggers reidentification risks?
Reidentification risks are triggered by combining quasi-identifiers (e.g., age, location) with external datasets or using advanced computational methods like AI-driven linkage attacks.